Obstacles to accelerated underwriting and the ‘3 C’s’ of data
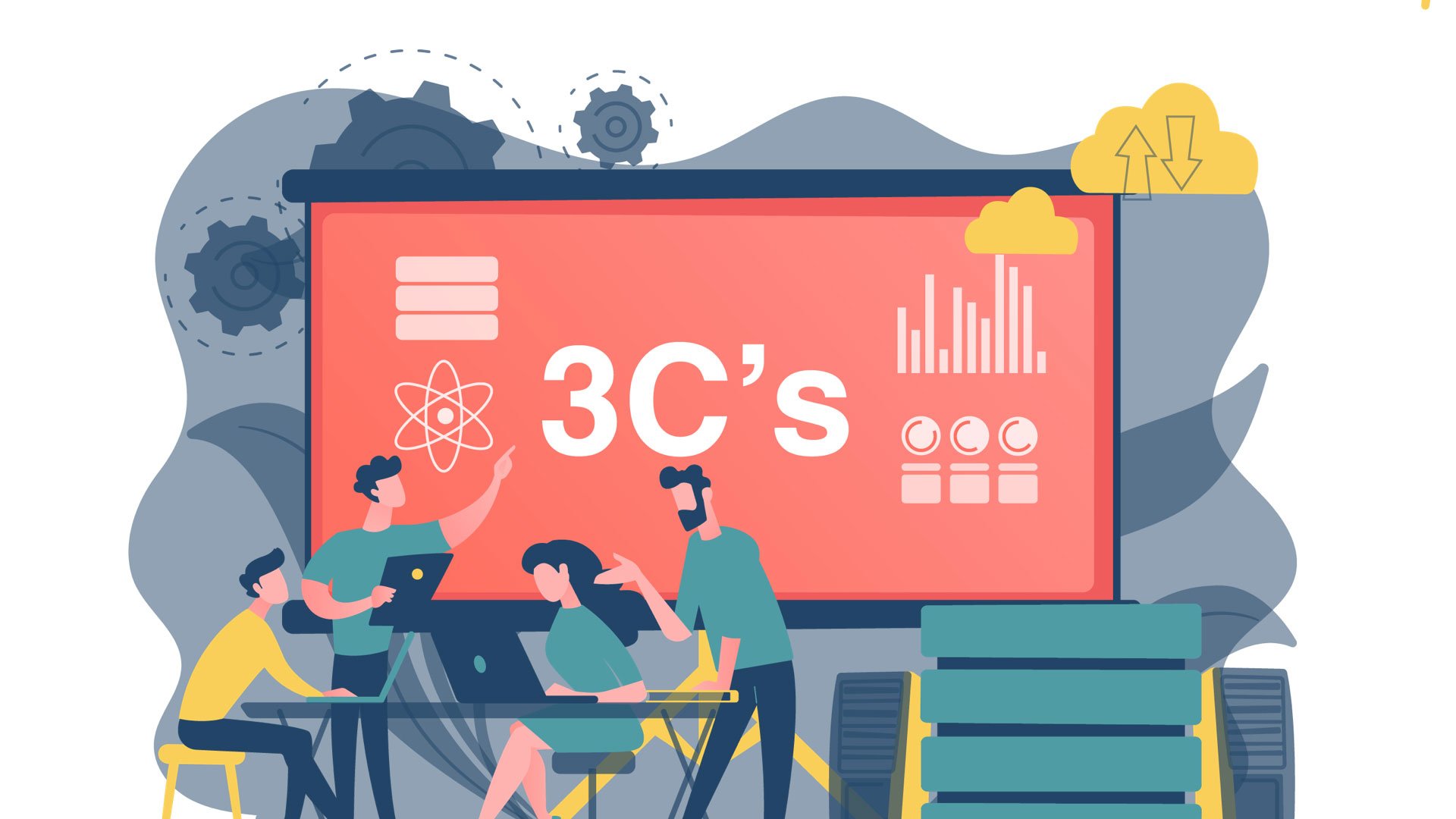
There’s a fact in the life insurance industry: The faster you can put an offer in an applicant’s hands, the more likely they are to purchase. So any tool that can speed the underwriting process with equivalent or better risk selection is highly attractive to the industry. It’s also one of the main reasons companies are scrambling to implement predictive models into their risk assessment — they can significantly accelerate the underwriting process, leading to quicker, more accurate risk assessments and a faster time to offer. What’s more, the characteristics of digital risk assessment are transparent, fair and “explainable” to applicants, advisers and agents.
The most important determinant of a predictive model’s speed and accuracy? Data. That is:
- Figuring out what data you need
- Collecting that data from applicants and/or purchasing it from a vendor
- Structuring that data so a predictive model can use it
Predictive risk models work best when your data adheres to the 3 C’s: consolidated, copious and converted. The 3 C’s exist in a perfect world, but who lives in that? Let’s take a closer look and examine some workarounds if you fall short.
Predictive risk models work best when your data adheres to the 3 C’s of data.
Consolidated
Getting the right data into a single easy-to-access location is important. If your data is spread across disparate systems in your enterprise systems (or outside of it), it should be consolidated into a holistic and well-designed data warehouse so it can be easily accessed by a risk model.
That warehouse, by the way, needs to be up to date. Data that resides in an obsolete legacy data warehouse can be difficult for a risk model to read and use.
Speaking of being up to date: While your data warehouse should be current, the data in it should be as well. It stands to reason: Fresh data is better than old data in order to ensure the effectiveness of the risk model’s output.
We realize, of course, that this is no small endeavor. It’s more likely an “always on” endeavor, which is why you should work with a partner that can accommodate the lack of consolidated data. You should be able to batch process records until your data warehouse is up to snuff. We help clients consolidate their data all the time … it’s what good partners do.
Copious
This shouldn’t be a surprise: The more data points you can feed into a risk model, the more accurate your underwriting will be. An important caveat, however, is that all data is not created equal; there’s a balance. Models such as LifeScore Med360 use 49 data points to assess mortality risk. We’ve done a considerable amount of analysis and chosen those particular data points for two reasons: They’re readily available from labs and they’re reliable indicators of risk. This means that if you only have 48 data points for your applicant, the assessment may not be as accurate.
Your goal is to have as much current, structured data as possible for your predictive model. That may mean obtaining some, most or all your data from third-party sources — most of you are already doing this. In our analysis, the cost of buying data is more than justified by the benefits gained from expediting risk assessment.
One of the biggest obstacles is the structure of your data...structured data works; unstructured data doesn't.
Converted
One of the biggest obstacles is the structure of your data. With predictive models, structured data works; unstructured data doesn’t. Even if your data is consolidated and up to date, it’s of little use to risk models if it’s not structured. Structured data — names, dates, addresses, credit card numbers, stock information, etc. — is highly organized and easily understood by machine language. It fits neatly within fixed fields and columns in relational databases and spreadsheets.
Structured data is also important for the “Explainability Factor” — the ability to identify the key data points that influence a risk model’s results and explain them to an agent, adviser or applicant. Another benefit of structured data is this: It’s useful for other company functions such as finance, reinsurance treaties and marketing. There is literally no downside.
Unstructured data such as text, paper applications, voice files, multimedia, freeform notes in medical records, etc., is not arranged according to a preset data model or schema. So it cannot be stored in a traditional relational database. It must be first converted to a structured format that can be read by predictive models.
Your to-do list
Optimizing your data to feed into a predictive risk model isn’t necessarily quick or easy. But just like when you are painting, it’s important to do this prep work. To increase the efficiency and competitiveness of the business, it’s imperative that you:
- Create a web-based digital portal that enables applicants and agents to submit Part 1 and Part 2 of the application electronically so that it comes into your data warehouse and underwriting ecosystem already structured. Seriously, when is the last time you filled out a paper form?
- Upgrade and update your data warehouse for 21st-century demands to intake and consolidate the new data points from different third-party providers.
- Convert your existing unstructured data into the structured format that risk models will accept.
- Combine your own in-house data with that provided by outside sources, such as applicants’ medical lab results, electronic health records, medical claims, motor vehicle records, credit information and so on, to provide a more holistic view of applicants’ health.
Sound a little daunting? Not gonna lie, it’s not easy to consolidate and convert your copious amounts of data. There are short-term workarounds and there are companies that can help. Third-party providers can supply data; you’re probably already using them. Plus, there are other companies that can structure unstructured data using techniques such as natural language processing. One large carrier digitized years of paper applications into its data set; now the company’s staff members can assess risk faster and more accurately than traditional underwriting.
To stay competitive, embrace the data — it is the most important element on the road to better and faster underwriting.
MM202512-303746