Life underwriting, data science and swiss cheese
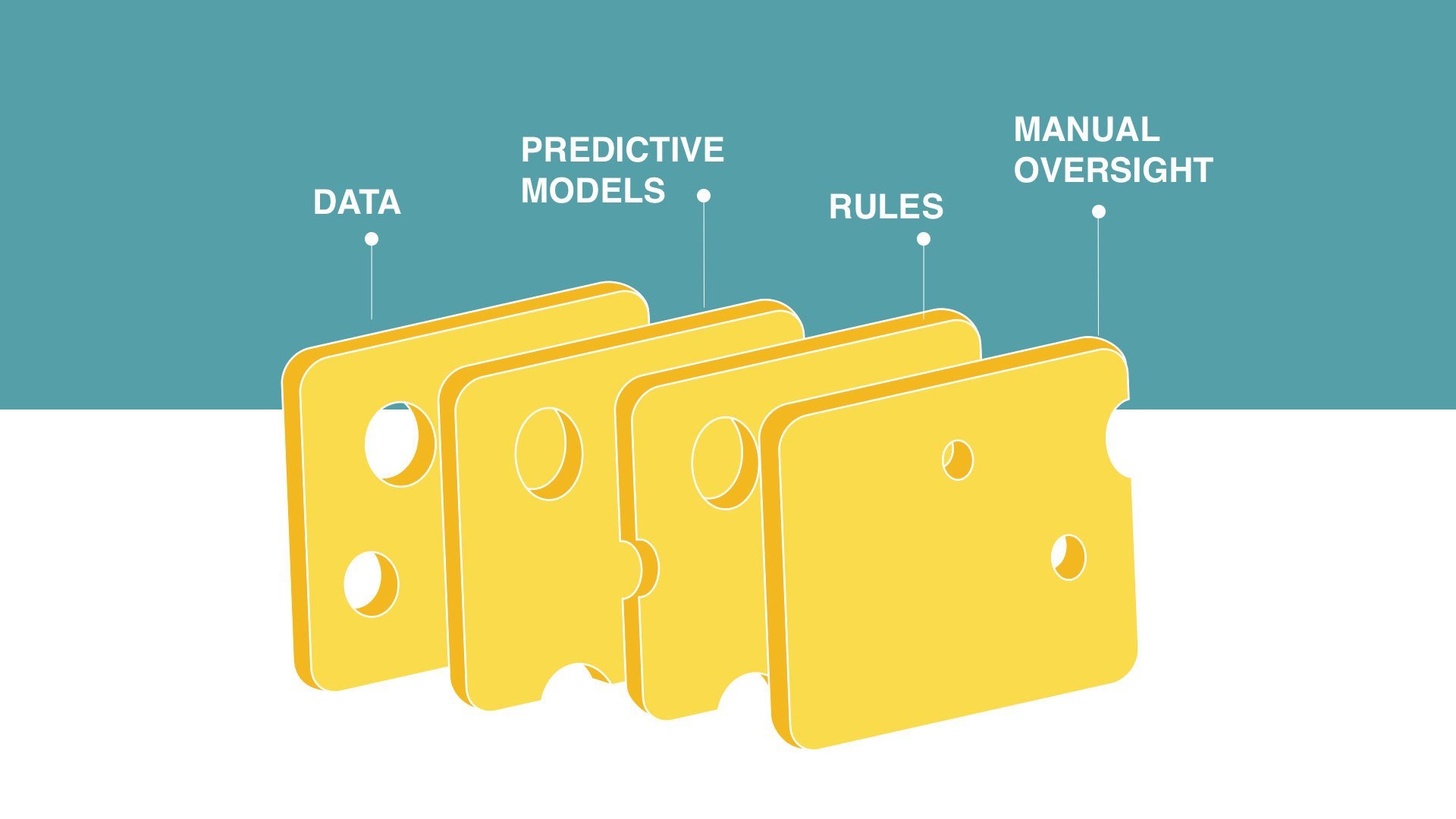
These seemingly unrelated items illustrate the progression of automated and accelerated underwriting and how data science is working to fix the holes.
It’s clear to any life insurance carrier or reinsurer that to compete in today’s digital-centric marketplace, the company must have a data science capability to help accelerate and automate the underwriting process. Some carriers try to build, manage, oversee and analyze every component of that capability in-house — and quickly find it to be time-consuming and costly. The time and cost can be justified with sufficient operational scale, but for everyone else, that approach doesn’t make sense.
Dean Regrut, head of product, along with panelists at the recent AHOU annual conference discussed some of the factors that companies should consider when deciding whether to build or buy various data science capabilities and deliverables, including predictive models.
Even the largest companies tend to underestimate the effort and cost involved in building and maintaining a predictive model.
The downside of inside
Some carriers will be tempted to build as much as possible themselves. Even the largest companies tend to underestimate the effort and cost involved in building and maintaining a predictive model.
While many companies can appreciate the cost and effort involved in building a proof-of-concept risk assessment model or natural language processing capability, they tend to underestimate the difficulty of getting a new model or service into production. Reinsurers and regulators need to be on board, and there are ongoing costs to maintain and monitor performance over time. The load on internal legal and regulatory affairs, data governance teams and data engineers is significant, not to mention the load on data scientists.
Before building, consider these five often-overlooked requirements:
1. The need for massive amounts of data
When you build a predictive risk model to support accelerated and automated underwriting, amassing the broad and deep trove of data required to build, train and test that model is one of the biggest challenges. The best models train on observed mortality rather than predicting prior underwriting decisions. In order to get a sufficiently large number of deaths into the population, you need hundreds of thousands of applicant records and a long-enough observation period so that a mortality pattern can emerge.
If your historical data isn’t sufficiently robust, you may not have enough observed deaths within your outcomes to produce a model that’s credible across all cohorts. You may be able to substitute outcomes with data from a partner or vendor, but that can be costly.
2. The ability to find and structure the data
Oftentimes, the elephant in the room is the state of the data. Solving for this issue takes both detective and grunt work. Once you find the right data in disparate and sometimes legacy systems (or from a third party if you don’t have it internally), it needs to be structured so that it is easily understood by machine learning — an often tedious but absolutely necessary task that makes the data meaningful in model development.
3. Ongoing monitoring and maintenance
Your accelerated roadmap should include constant monitoring to identify the holes in the process. Use the resources at your disposal to fix them. We’ve previously written about how models and rules work better together. Rules can help fill the holes in tandem with additional predictive solutions and your team of underwriters, actuaries and data scientists.
4. An experienced team of data scientists
In a business world that runs on data, data scientists are in high demand.
It’s difficult to build a sophisticated, agile team of design-thinking data scientists, even when the market is awash with them. Today, qualified data scientists are rare, and the labor market is expected to be tight for years to come. Add the recent explosion of artificial intelligence and generative AI capabilities — and the data science teams required to harness them — and insurance carriers face real challenges in cobbling together such internal teams.
5. Sufficient budget
Any carrier that’s begun the process of building a formidable data science capability will tell you it’s not cheap. And in tight economic times, not many IT budgets can accommodate from-the-ground-up construction of a data science capability. A combination build-and-buy approach enables you to judiciously select which capabilities are most cost-effective to take in-house and which to outsource.
Models are efficient and accurate when applied properly, rules can be restrictive and manual oversight is expensive and slow. In any approach, some risks will sneak through.
The Swiss cheese approach
There is no one silver-bullet solution as you look to digitize as much of your underwriting as possible. Predictive models work within a rules environment and with appropriate manual oversight. Models tend to be efficient and more accurate when applied properly. Rules tend to be overly restrictive and therefore reduce operational efficiency, but they add protective value. Manual oversight is relatively expensive and slow.
Getting the right combination of data and predictive models to complement your rules and manual oversight is tricky. In any approach, some risks will sneak through; each layer that is added to mitigate risk is like a piece of Swiss cheese. With enough layers, you can cover all the holes but each layer has a cost.
The question is not whether you invest in data science or not, but rather how to best apply this scarce resource. Data scientists need to continually monitor model results and identify each hole where risk is slipping through. They also need to determine whether to build or buy the next layer of cheese. With such a precious resource, apply it where it brings the most value.
Play to your strengths, outsource the rest
In sum, why try to invent what’s already been invented? Just as manufacturers outsource the components and systems that they’re unable or unwilling to build internally, insurers and reinsurers should buttress their own data science capabilities by leveraging the considerable external resources that can help them to fill the gaps.
A similar topic was discussed as a panel presentation on April 19 at the 2023 AHOU Annual Conference. Panelists included:
Dean Regrut, Moderator, Head of Product, LifeScore Labs
Brian Lanzrath, Director of Analytics, ExamOne
Dan Brown, Chief Underwriter, Baltimore Life
Katherine McLaughlin, VP, Data Analytics, SCOR
David van Caster, Senior Director, UW Analytics, Northwestern Mutual