Why insurers are struggling to build a data science capability
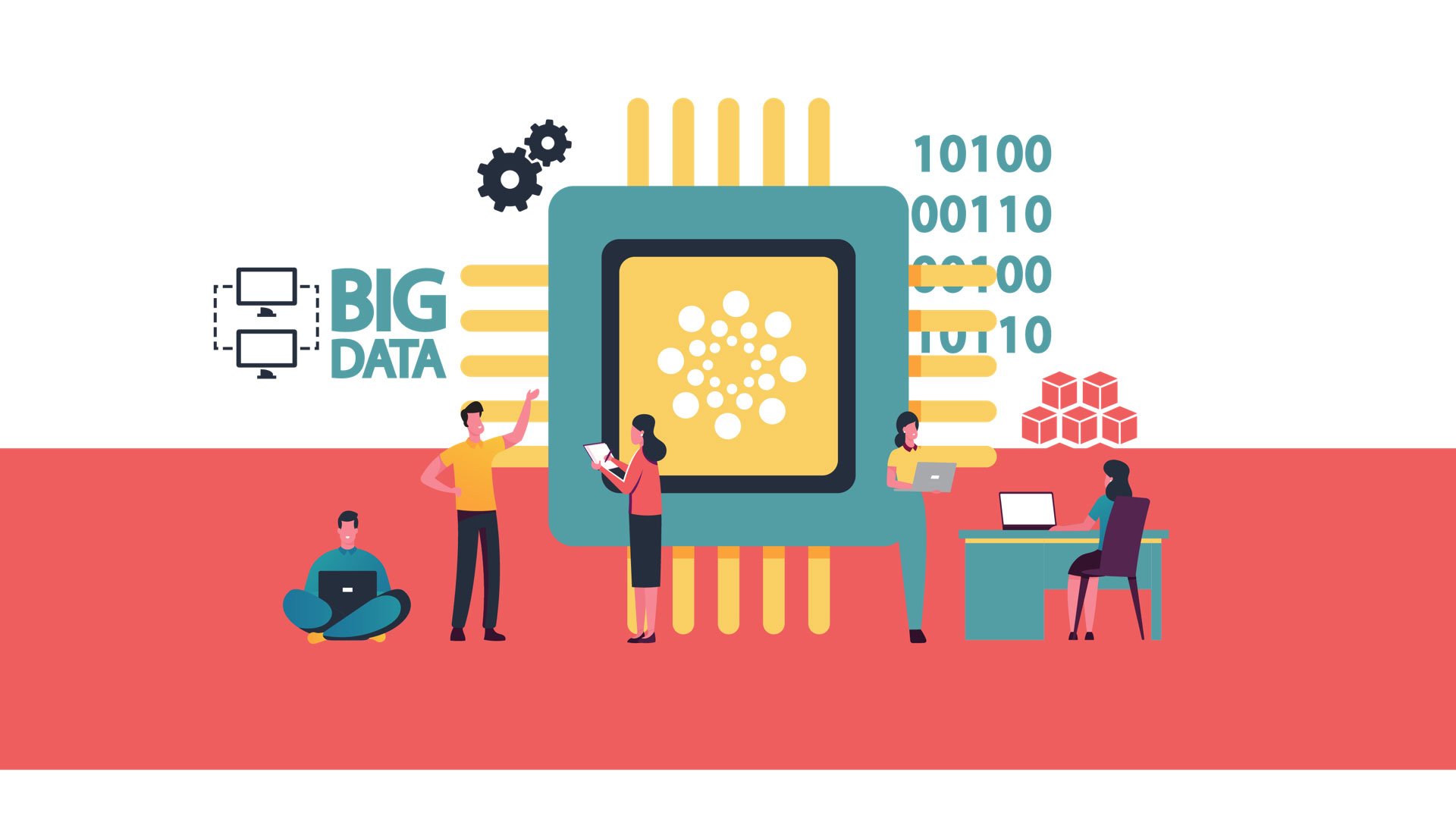
Data and data scientists are required ingredients in the transformation of the life insurance industry. Data scientists are, however, in short supply. The insurance industry is competing with companies like Google, Apple, Meta and others in the battle for data science talent — but we have a solution.
In the life insurance industry, the use of data-driven predictive risk assessments and associated predictive risk models is undeniable, expanding rapidly and gaining significant traction.
It’s easy to see why: data-driven risk models enable life underwriters to measure risk much more accurately, consistently and quickly. For this reason, underwriters have embraced the growing and transformative role of data science in mortality risk assessment, and insurers benefit from more accurate risk assessments than were possible just a few short years ago.
An important reason that a data science–driven predictive model is so effective in enhancing the traditional underwriting process is its unbelievable processing power. While traditional analytical methods can generally factor in two or perhaps three data points at a time (e.g., BMI and blood pressure), a predictive model uses machine learning to identify patterns and complex interactions among literally dozens of different lab and health variables.
Given the obvious power of data science in risk selection, carriers may be tempted to build their own internal capabilities. But there’s a problem.
Where are the data scientists?
One of the biggest challenges for life insurance carriers is that leveraging data science requires hiring data scientists. And those professionals are in very short supply. According to Owen Hughes, Senior Editor of zdnet.com, there is currently a shortage of 150,000 to 200,000 hardcore data science professionals worldwide.
“Employers are desperate for data scientists as demand booms,” Hughes writes. “The demand for data science is growing fast, yet recruiters are struggling to find data scientists that can help them move their digital ambitions forward.”
A recent report by the U.K.’s Royal Society warns that demand for data scientists and data engineers has more than tripled over five years, leaving businesses “crying out for professionals to unlock the potential of new technologies” such as machine learning and artificial intelligence.
Implement a workaround
It’s difficult to build a technologically sophisticated, agile team of design-thinking data scientists even when the market is awash in them. Qualified data scientists are rare, and the labor market is expected to be tight for years to come. Insurance carriers will face real challenges in cobbling together such an internal team.
Enterprising carriers have found a work-around: buy instead of build.
Fortunately, carriers today have access to the benefits of data science without needing to build a team of data scientists. Enterprising carriers have found a work-around: buy instead of build. This approach circumvents the need to develop one’s own team and predictive model. And in many cases, it gives carriers access to models that are trained on larger, more complete data assets than most have in-house.
Working with a vendor that already has a proven model saves months if not years of building a team and developing a solution. Plus, experienced data scientists have protocols in place to ensure that your data is safe and handled responsibly and ethically given its highly sensitive nature. And just as important, a vendor’s data science team is willing, capable and typically contractually obligated to customize each predictive model to a carrier’s unique underwriting appetite and needs.
Build your own? Not necessarily
Of course, carriers are concerned about outsourcing risk assessment, arguably one of the most proprietary and important elements of their business. For insurance executives, the temptation to own this capability is natural, but is it necessary?
If you were building a life insurance carrier from scratch, which capabilities would you build internally? Which would you outsource?
We typically see new entrants invest in marketing and customer acquisition, and rely on third-party platforms for the underwriting infrastructure and risk assessment tools. This approach enables carriers to be more agile and competitive on price and still leverage the latest data and models available from a variety of vendors.
Driving digital transformation
Using advanced predictive analytics tools like artificial intelligence and machine learning, third-party data scientists craft the kinds of predictive models that are driving digital transformation in today’s life insurance industry — solutions such as accelerated and lab-based risk models that seamlessly integrate with any workflow, rules engine and/or underwriting platform.
The insurers that outsource data-driven risk assessment gain a considerable competitive advantage.
The insurers that outsource data-driven risk assessment gain a considerable competitive advantage; others that go it alone and build their own are in danger of falling behind.
For insurance carriers, then, there are two paths: try to build an internal data science capability over time, with all the incumbent fits, starts and wrong turns, or partner with a leader in the space that has already developed superior data science talent and solutions.
The choice seems clear.
MM202508-302615