How to fall in love with a (predictive) model
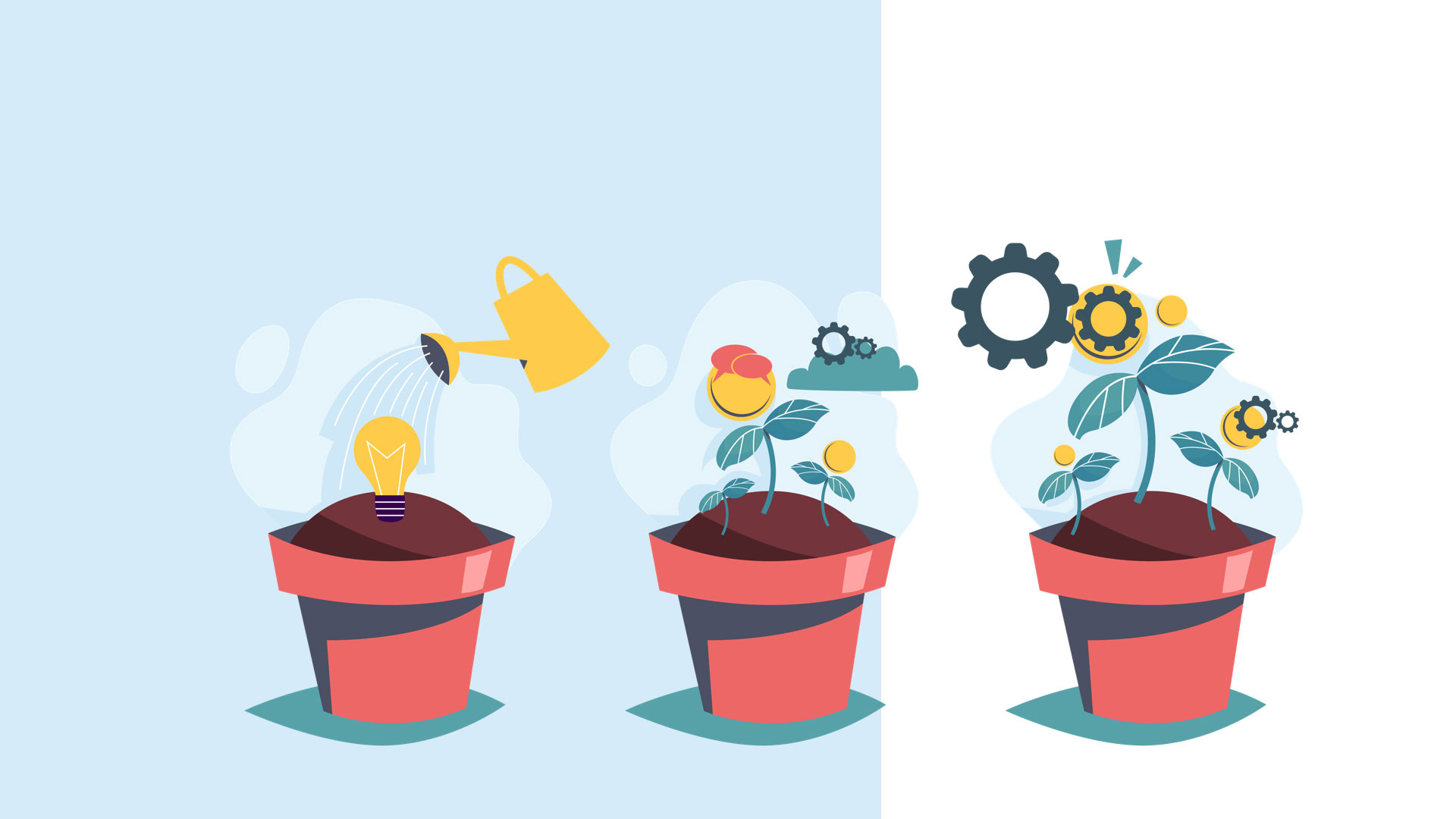
Today’s life insurers are working to digitize as much of their underwriting as possible. As they do, many are struggling to determine when to use human judgment, rule sets and predictive models. What’s the optimal structure within their underwriting ecosystem?
Many insurers and reinsurers work using a large, long-standing ecosystem of rules they’ve come to trust, and they’re reluctant to cede any part of those systems to a data-driven risk model. The underlying fear of the unknown suggests that if one or two existing rules were to be displaced, higher-risk applicants could slip in through the resulting gaps.
Deep down, most insurers are aware that a model performing some underwriting functions can be more efficient and effective than rules that govern a gate. They’ll also admit that many of their existing rules may be outdated and inefficient. Often, rules have been slowly spackled on top of each other over time, and that they may duplicate and reduplicate each other.
In spite of any initial reticence, forward-thinking insurers are realizing that when today’s risk models are compared to traditional human-created rule sets, the efficiencies provided by these models are more than worth the effort.
In order to trust a complex model, you need to trust the data science team that controls the production of the model.
Letting go of control and learning to trust
Why do some insurers hold so tight to rule sets? It’s all about control and explainability. With human-created rule sets, you maintain complete control over what defines a rule or underwriting decision. With a model, some of that control shifts to the data scientists who create the model. In order to trust a complex model, you need to trust the data science team that controls the production of the model.
With humans and rules, you have a log of explainability, enabling you to trace every step in the decision path that leads to the final rating decision. With a model, you forfeit some of explainability because at least a portion of the model’s computation is opaque. The best model implementations include interpretability mechanisms which can help underwriters understand some of that opaque logic, but they don’t perfectly translate the full complexity of a model’s internal logic.
...human and rules-based decision making is linear and limited, while a model’s decision-making is nonlinear and unlimited.
The advantages of risk models far outweigh the drawbacks. The primary reason is this: human and rules-based decision making is linear and limited, while a model’s decision-making is nonlinear and unlimited. In other words, predictive models can encompass and process an infinitely larger set of data points than humans can. A risk model easily manages highly complex mathematical relationships between the variables that determine risk. And the result is that models have been proven to make better, more accurate risk assessments.
Another advantage models have over traditional underwriting is time. With humans and rules, there’s a significant amount of underwriter time and working hours are spent painstakingly combing through data to make decisions. In contrast, a model’s processing time is virtually instantaneous. So rather than devoting precious hours to a manual underwriting process that uses a series of rule sets, carriers and reinsurers that implement predictive models into their underwriting can spend those hours intelligently assessing a model’s output and making adjustments or evaluating those applicants that require human logic. That translates to better risk assessment and the ROI of faster underwriting decisions.
Where does a model fit?
So, where should you deploy a risk model in your own underwriting process? Consider your entire process or ecosystem and think about the types of efficiencies you want to gain at certain stages in that ecosystem.
Use Case 1
Do you want faster decision-making? Consider a risk-assigning model that deploys after you receive the first influx of data from an applicant.
Use Case 2
Still don’t have enough data to make a final decision? Consider implementing a model to triage risks at a specific juncture and separate people into similar paths rather than using models to make a final say.
Use Case 3
Do you want to improve mortality outcomes—that is, better stratify risk? Consider putting a model at the back end of the process, after you’ve received all data (blood work, labs, etc.).
Download the Use Case Infographic
The importance of the pilot (a practice date)
Want to dip your toe into the risk-model waters before diving in? Pilot the model and then evaluate the results before making any change to your UW ecosystem and processes.
Remember: Wherever you choose to deploy a risk model, the change might feel a little unsettling at first. But a model makes nonlinear connections swiftly and deftly, and can help improve speed, efficiency and risk assessment at whatever point in the process it’s inserted.
Carriers we work with begin by implementing a model to address a specific inefficiency in their process. They start small, evaluate the results, and then tackle the next pain point. That slow, steady and strategic implementation is what builds trust in the model. That trust makes it easier for underwriters to loosen a little of their control over the process and may even lead to the beginnings of a crush.
We’re confident that love will come in time.